More focus and desire to finding answers, and less hype
My view on why newcomers should focus on the basics and on the true meaning of working with data
My view on why newcomers should focus on the basics and on the true meaning of working with data
If you ask me what’s the most common question asked in the industry, I’d say that it is “how to become a data scientist.” It kind of makes sense, right? With all the hype, news, and glorification of the field, it is reasonable to have many prospects interested in basking in the grandeur of data.
Go to Google, type this trendy query and upon clicking enter, you are bound to receive a page with thousands of links, pointing to many, way many, guides, resources, tutorials and whatnot.
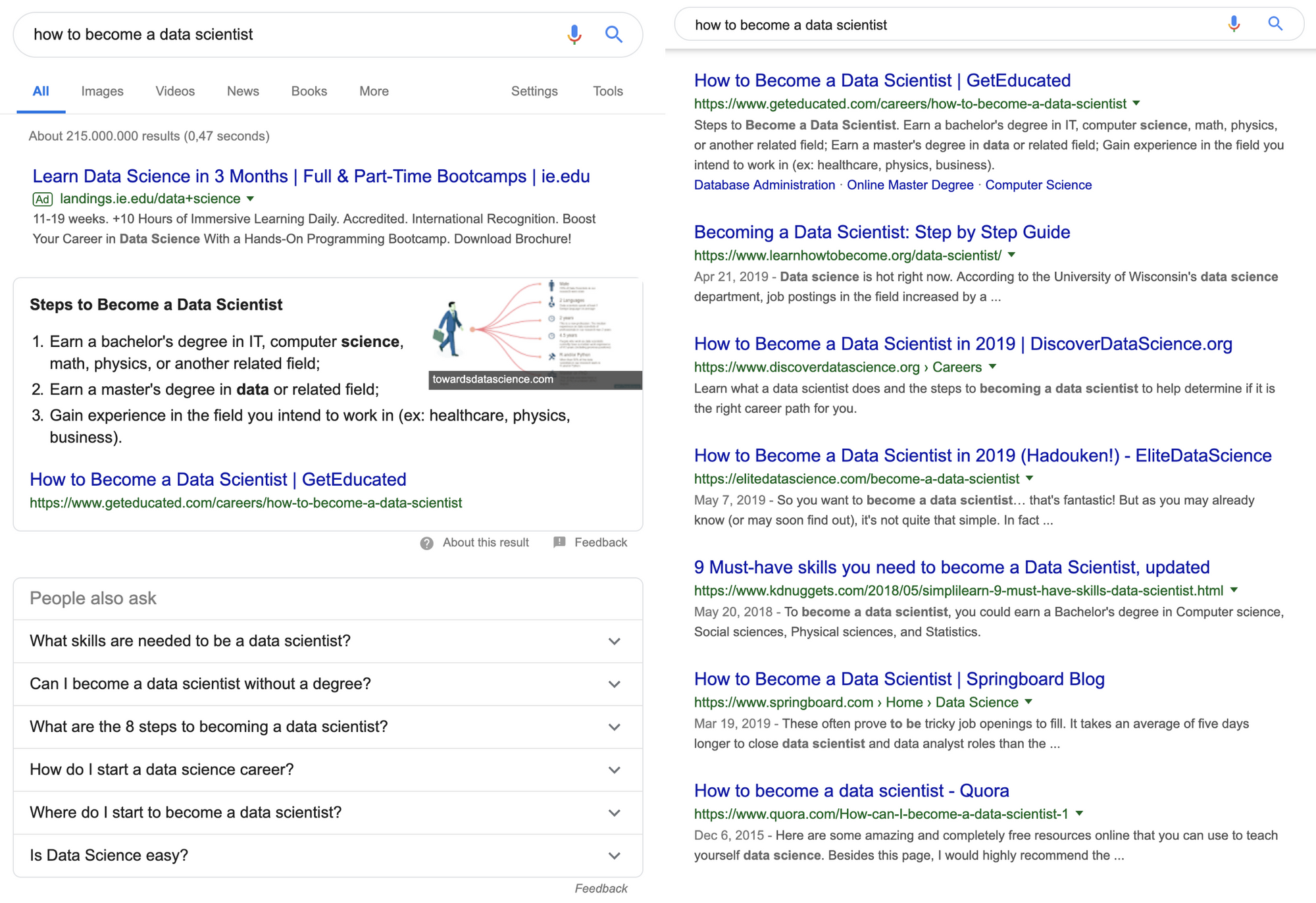
And this could be a problem for newcomers.
Comments and instructions such as “you should learn this library”, “master this framework”, “become an SQL God”, “be a Kaggle Master”, “recite all the different activation functions”, “have dinner with Yann LeCun”, and so on, and some of the lines you might find (maybe not the last one).
The amount of information out there can be overwhelming! So overwhelming to the point where the newly interested people will give up. Want further evidence? This Reddit thread, titled “Does anybody else feel overwhelmed looking at how much there is to learn?” presents some example of this.
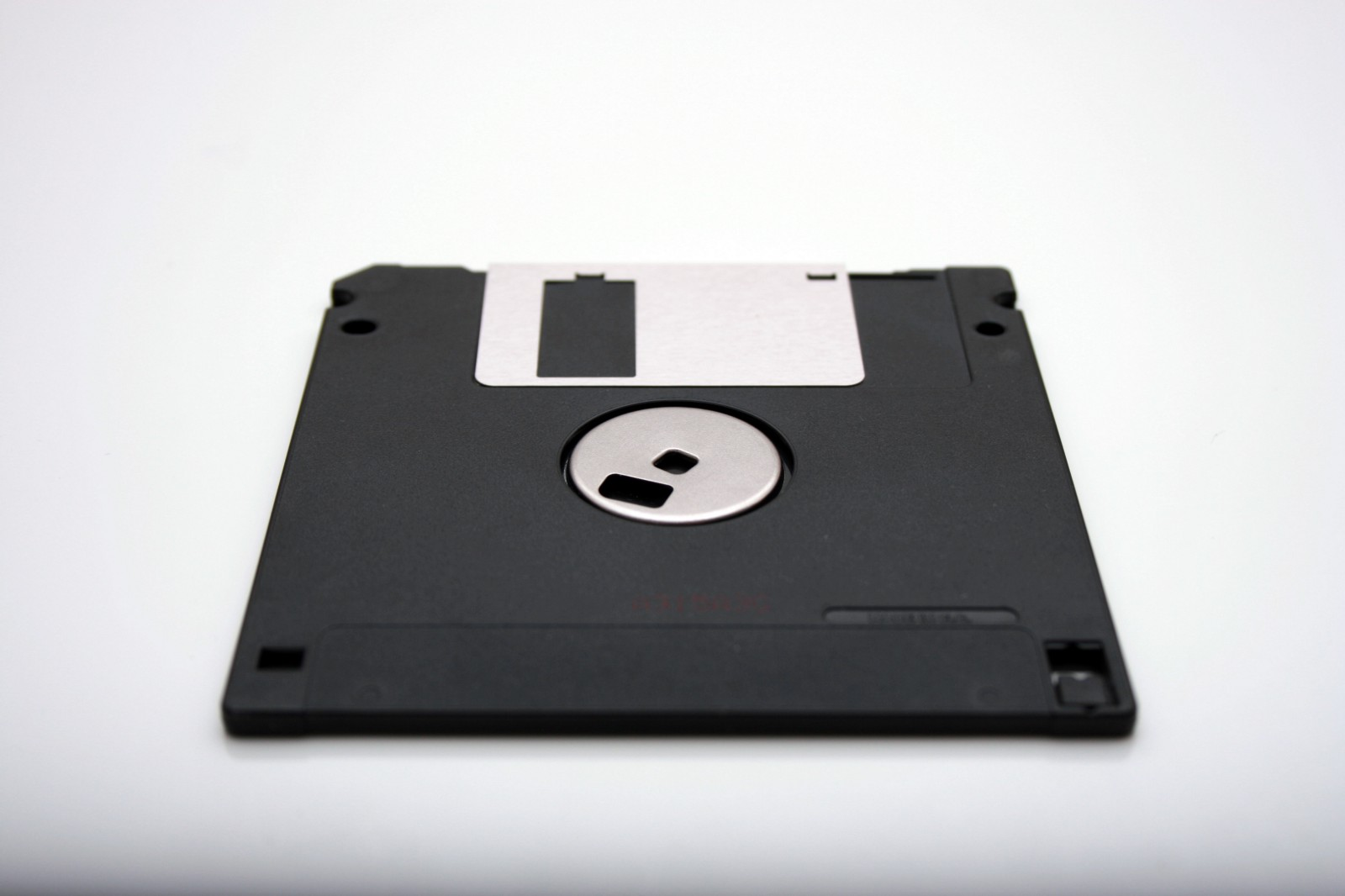
However, what if instead of throwing these toddles into the wild and letting them survive on their own, we, the community, instructors, mentors, friends, and leaders, could soften the fall and change the way we are introducing these novices into the field of data.
If I were to start today my decision to learn the basics of data science and ask Google how to become a data scientist, I’d probably close my browser, and cry under my desk. For starters, the term “data science” is broad, and it encompasses things I have no idea they exist. Let’s take a look at this.
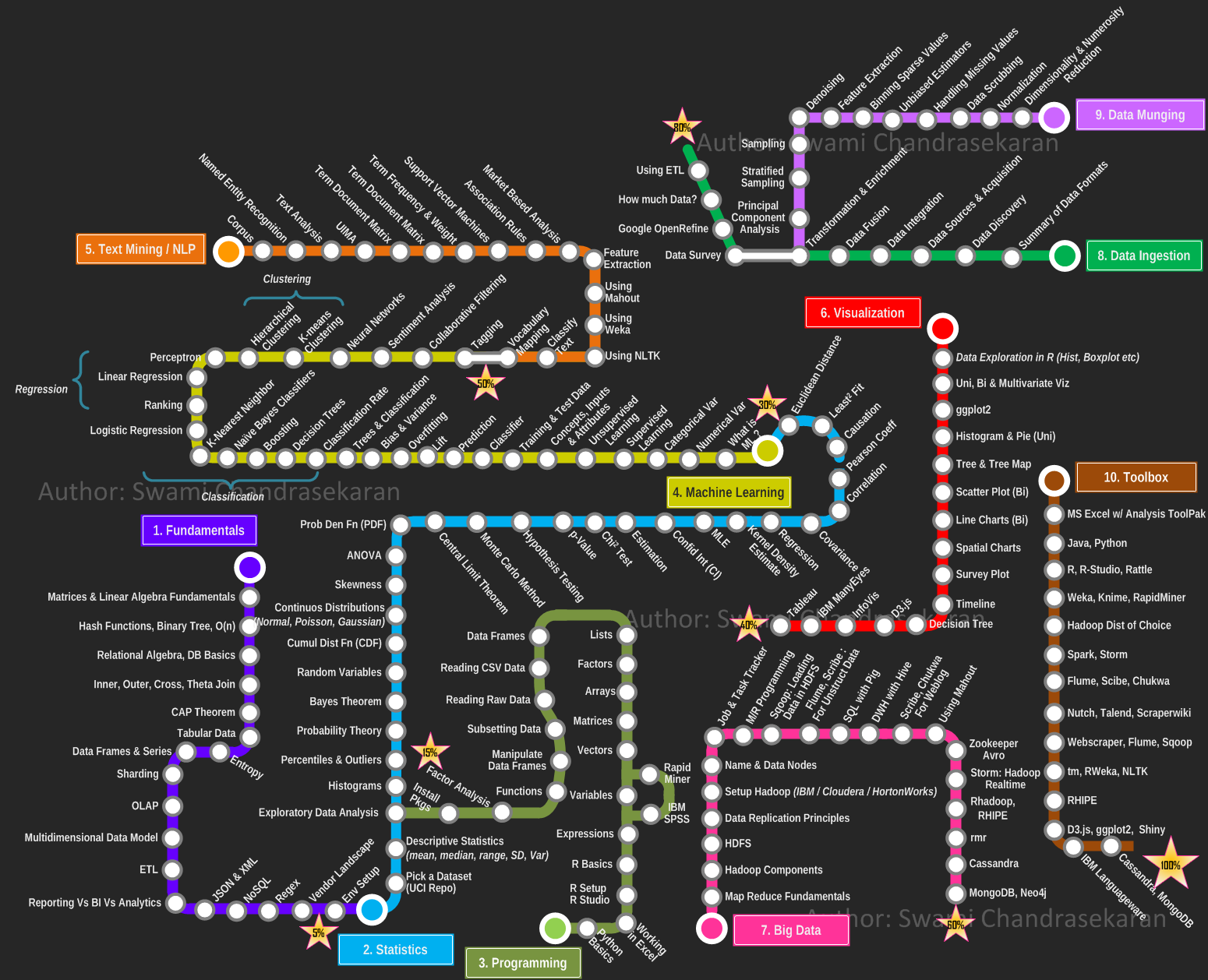
See this? It’s a lot! How is this supposed to motivate someone? Moreover, what I see here — and this is my opinion– is some sort of roadmap and a checklist with arbitrary completion percentages that are supposed to state how much of a data scientist you are.
Sidenote: this image is from 2013, and some of the technologies presented here are outdated. Also, I’m not saying the picture is wrong; it provides very valuable information on how to get started. What I wanted to illustrate is it might cause some frustration to someone who wants to get its hand dirty with data.
In reality, and this is what I have learned during my career, if someone’s title is “data scientist,” I’m sure that their day to day tasks or daily business is about one particular topic, for example, risk assessment, churn analysis, data visualization, being an SQL God, and so on.
Which brings me to my first point.
What if instead of suggesting all this vast amount of information, websites and “how to become a data scientist” guide to the newcomer, we try to pinpoint or at least approximate, what exactly prompted and motivated them to join our wonderful world of data. Was it data visualization? Or maybe machine learning? Love for statistics?– if the answer to this question is either money or hype, good luck.
By doing so, by asking this simple question, then we could guide, and orient them towards the right thing. Once the person is in this path and has obtained a clear understanding of the basics, then it’s up to them to decide how to complement this freshly acquired knowledge.
Now you might ask: “this is nice and everything, Juan, but how.” Good question, and yes, it is not an easy task. But, did you notice how I use the pronoun “we”? By that, I meant us, the community, and mentors I mentioned a couple of paragraphs above. Therefore, my message here is that if you, someone who is currently in the industry, is ever in the position of helping, mentor, tutor, someone, please consider doing it. I’m sure they’ll appreciate it.
The second point I want to bring to the table is that regardless of the chosen field of work, we should always make it clear that the ultimate goal of a data person is to find answers to questions. I believe, and again, this is my opinion, that amid the chaos of the hype, libraries, fancy methods and such, we have lost track of the essence of the field. This lack of focus is somehow understandable, and it even happens to me sometimes. We keep seeing all these new toys being release, and we want to implement them right away and to get the best accuracy scores. Nonetheless, let it be clear that at the end of the day, your boss and enterprise wants to know, how much money the company will gain or lose today, which tone of green is better, or whether the user is a spammer or not.
So please, next time someone asks you what’s the key to being a data scientist is, try to include the words “question” and “answers” in your response.
And to the beginners, take it one step at a time, and keep in mind that no one knows everything.
Thanks for reading.